Vol. 2 No. 01 (2025): Student Dropout Prediction using DeepFM Algorithm
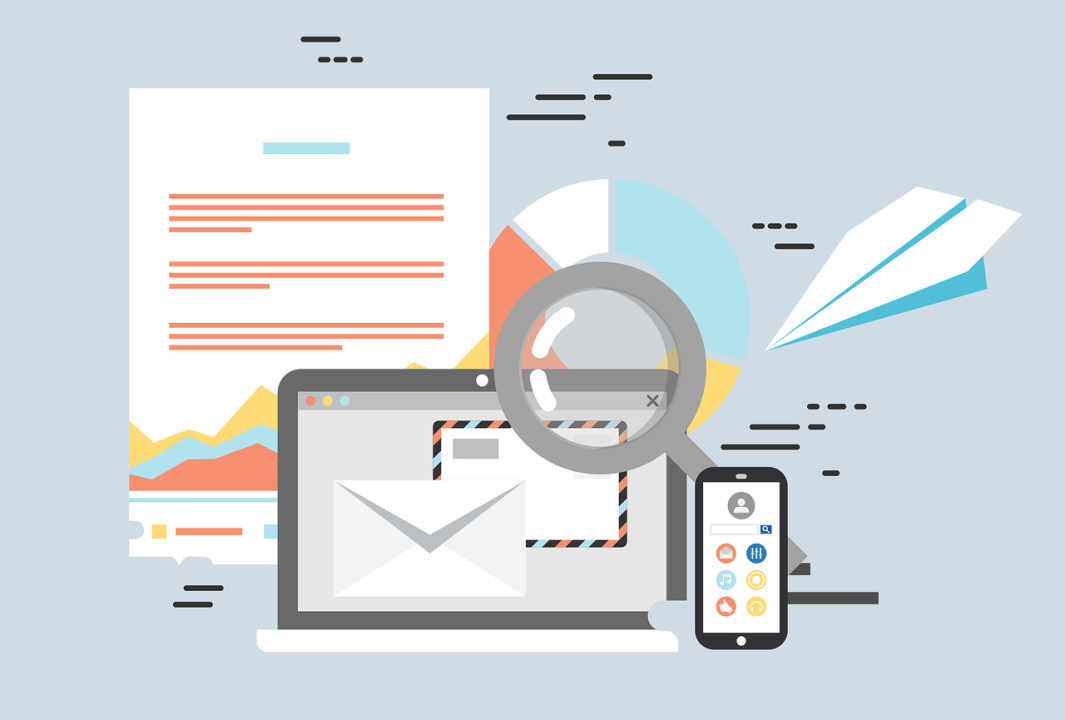
Abstract
This project revolves around the development and implementation of a predictive model for anticipating student dropout utilizing the innovative DeepFM algorithm. DeepFM, a fusion of deep learning and factorization machines, proves to be a potent tool for unraveling complex patterns within diverse datasets. The project aims to leverage the algorithm's capability to analyze and interpret multifaceted features such as academic performance, attendance, and socio-economic factors. Through the integration of deep neural networks and collaborative filtering, DeepFM demonstrates proficiency in discerning intricate relationships, contributing to accurate student dropout predictions. The project's primary objectives include the exploration of DeepFM's suitability for handling sparse and high-dimensional student data, as well as its potential to offer proactive insights for educational institutions to address and mitigate dropout risks. The anticipated outcome is a robust predictive model that aids educational institutions in identifying potential dropout candidates, facilitating timely interventions and support mechanisms to enhance student retention.
Index Terms
Predictive modeling, Student dropout prediction, DeepFM algorithm, Deep learning, Factorization machines, Multifaceted features, Academic performance, Attendance, Socio-economic factors, Deep neural networks, Collaborative filtering, Sparse data handling, High-dimensional data, Dropout risk mitigation, educational institutions, Timely interventions, Support mechanisms, Student retention, Data analysis, Machine learning applications.