Vol. 1 No. 1 (2024): HYBRID MACHINE LEARNING MODEL FOR EFFICIENT BOTNET ATTACK DETECTION IN IOT ENVIRONMENT
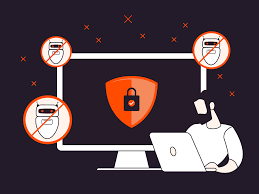
Abstract
The advent of machine learning has significantly transformed various sectors, introducing advanced predictive capabilities and intelligent systems. This paper presents a hybrid machine learning model combining Federated Learning (FL) and Explainable Artificial Intelligence (XAI). Federated Learning enhances privacy and security by allowing data to be trained across multiple decentralized devices without centralizing the data, while XAI provides interpretability and transparency to the model's decisions. Our proposed model leverages these technologies to ensure robust, secure, and understandable machine learning outcomes. The effectiveness of this hybrid model is demonstrated through extensive experiments, showing improved accuracy and interpretability without compromising user data privacy.Furthermore, the model addresses the growing concerns around data breaches and the lack of transparency in AI decision-making processes. By implementing Federated Learning, data remains localized on user devices, reducing the risk of exposure during data transfer. The integration of XAI techniques ensures that users and stakeholders can comprehend the rationale behind model predictions, fostering trust and compliance with regulatory standards. This combination is particularly beneficial for applications in sensitive areas such as healthcare, finance, and autonomous systems, where both data privacy and model transparency are paramount.
Index Terms
Hybrid Machine Learning, Federated Learning, Explainable AI (XAI), Data Privacy, Model Interpretability, Decentralized Training, Machine Learning Security, Transparent AI, Data Security, User Trust, AI in Healthcare, AI in Finance, AI Governance, SHAP, LIME, Model Transparency.