Vol. 2 No. 04 (2025): Deep Learning-Powered Automated Detection of Abnormalities in Chest X-Rays
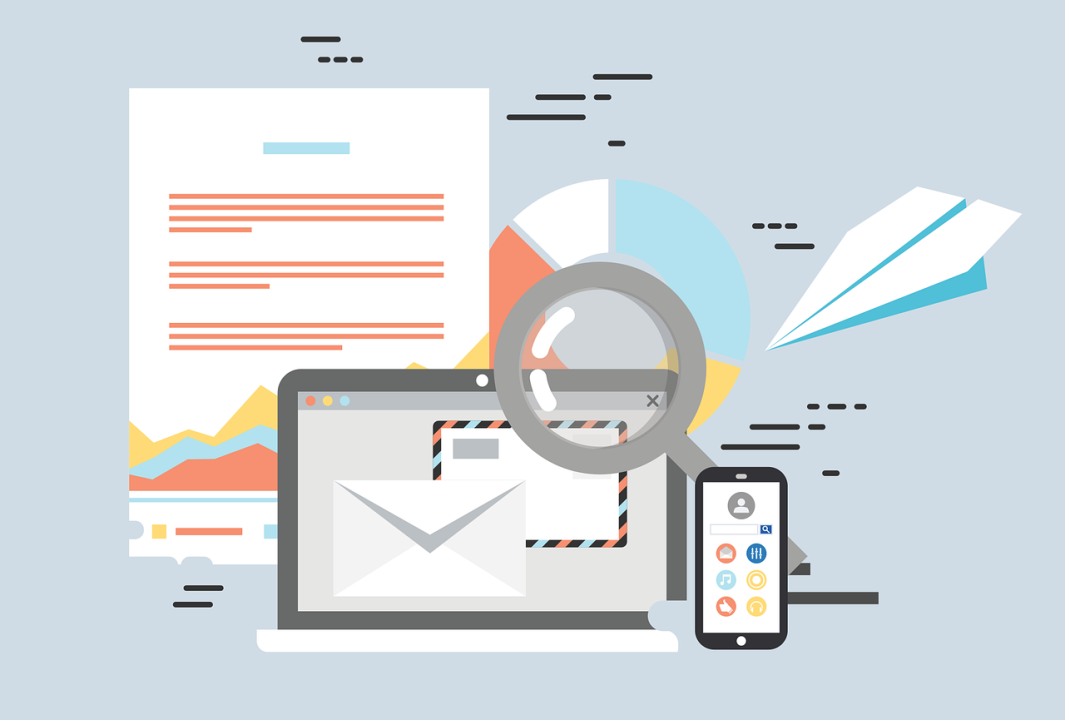
Abstract
Medical imaging plays a crucial role in diagnosing various diseases and abnormalities within the human body, with chest X-rays being one of the most commonly used modalities. In recent years, deep learning techniques have shown remarkable promise in automating the analysis of medical images, including the detection of abnormalities in chest X-rays. This project aims to explore the application of deep learning algorithms, particularly convolutional neural networks (CNNs), for the automated detection of abnormal findings in chest X-rays. The project will involve the collection and preprocessing of a diverse dataset of chest X-ray images, encompassing both normal and abnormal cases. Subsequently, deep learning models will be trained, validated, and fine-tuned using the collected dataset to accurately classify chest X-rays as either normal or abnormal based on the presence of various pathologies such as pneumonia, lung nodules, or pleural effusion. The performance of the developed models will be evaluated using standard metrics such as accuracy, sensitivity, specificity, and area under the receiver operating characteristic curve (AUC-ROC). The outcomes of this project aim to contribute to the advancement of computer-aided diagnosis systems in healthcare, potentially aiding clinicians in making more accurate and timely diagnoses, thus improving patient outcomes.
Index Terms
Medical Imaging, Chest X-rays, Deep Learning, Convolutional Neural Networks (CNNs), Automated Detection, Abnormal Findings, Dataset Collection, Preprocessing, Pathologies, Pneumonia, Lung Nodules, Pleural Effusion, Performance Evaluation, Accuracy, Sensitivity, Specificity, Area Under the Receiver Operating Characteristic Curve (AUC-ROC), Computer-Aided Diagnosis Systems, Healthcare, Patient Outcomes.